Documents
Presentation Slides
Partially observable model-based learning for ISAC resource allocation
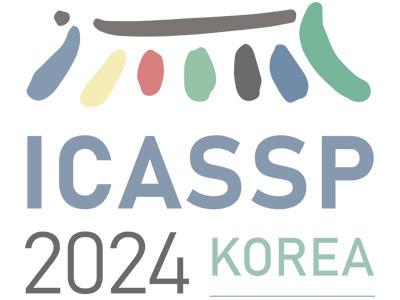
- DOI:
- 10.60864/z7d1-yx59
- Citation Author(s):
- Submitted by:
- Petteri Pulkkinen
- Last updated:
- 6 June 2024 - 10:27am
- Document Type:
- Presentation Slides
- Document Year:
- 2024
- Event:
- Presenters:
- Petteri Pulkkinen
- Paper Code:
- 3848
- Categories:
- Log in to post comments
This paper considers resource allocation problems for integrated sensing and communications (ISAC) systems operating in dynamic shared spectrum scenarios. Specifically, the paper proposes a new Model-Based Online Learning (MBOL) method that accounts for partial observability caused by noisy observations. First, the approach converts the partially observable Markov decision process (POMDP) to the equivalent belief state Markov decision process (MDP). Then, the state prediction model is learned from the sensor observations. A loss correction approach is introduced to solve the model learning problem under partial observability. The proposed approach is evaluated in allocating subcarriers and their powers to communications and sensing tasks in multicarrier ISAC systems. The simulations demonstrate improved performance in partially observable settings.