Documents
Presentation Slides
PEER COLLABORATIVE LEARNING FOR POLYPHONIC SOUND EVENT DETECTION
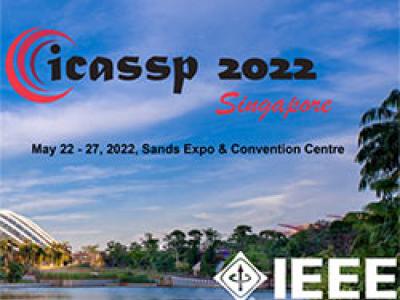
- Citation Author(s):
- Submitted by:
- Hiromitsu Nishizaki
- Last updated:
- 11 May 2022 - 8:13pm
- Document Type:
- Presentation Slides
- Document Year:
- 2022
- Event:
- Presenters:
- Hiromitsu Nishizaki
- Paper Code:
- AUD27.6
- Categories:
- Log in to post comments
This paper describes how semi-supervised learning, called peer collaborative learning (PCL), can be applied to the polyphonic sound event detection (PSED) task, which is one of the tasks in the Detection and Classification of Acoustic Scenes and Events (DCASE) challenge. Many deep learning models have been studied to determine what kind of sound events occur where and for how long in a given audio clip. The characteristic of PCL used in this paper is the combination of ensemble-based knowledge distillation into sub-networks and student-teacher model-based knowledge distillation, which can train a robust PSED model from a small amount of strongly labeled data, weakly labeled data, and a large amount of unlabeled data. We evaluated the proposed PCL model using the DCASE 2019 Task 4 dataset, and achieved an F1-score improvement of about 8.2 points compared with the baseline model.