Documents
Presentation Slides
Presentation Slides
PERFORMANCE ANALYSIS OF DISCRETE-VALUED VECTOR RECONSTRUCTION BASED ON BOX-CONSTRAINED SUM OF L1 REGULARIZERS
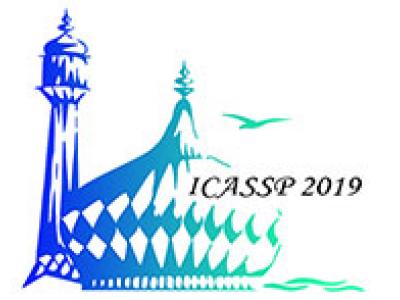
- Citation Author(s):
- Submitted by:
- Ryo Hayakawa
- Last updated:
- 15 May 2019 - 5:52pm
- Document Type:
- Presentation Slides
- Document Year:
- 2019
- Event:
- Presenters:
- Ryo Hayakawa
- Paper Code:
- SPTM-L3.04
- Categories:
- Log in to post comments
In this paper, we analyze the asymptotic performance of a convex optimization-based discrete-valued vector reconstruction from linear measurements. We firstly propose a box-constrained version of the conventional sum of absolute values (SOAV) optimization, which uses a weighted sum of L1 regularizers as a regularizer for the discrete-valued vector. We then derive the asymptotic symbol error rate (SER) performance of the box-constrained SOAV (Box-SOAV) optimization theoretically by using convex Gaussian min-max theorem. Simulation results show that the empirical SER performances of Box-SOAV and the conventional SOAV are very close to the theoretical result for Box-SOAV when the problem size is sufficiently large.