Documents
Presentation Slides
PLUG-AND-PLAY IMAGE RECONSTRUCTION MEETS STOCHASTIC VARIANCE-REDUCED GRADIENT METHODS
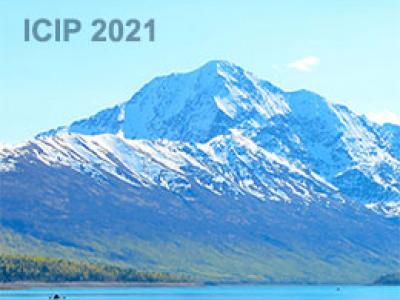
- Citation Author(s):
- Submitted by:
- Vincent Monardo
- Last updated:
- 23 September 2021 - 5:19pm
- Document Type:
- Presentation Slides
- Document Year:
- 2021
- Event:
- Presenters:
- Vincent Monardo
- Paper Code:
- 2542
- Categories:
- Keywords:
- Log in to post comments
Plug-and-play (PnP) methods have recently emerged as a powerful
framework for image reconstruction that can flexibly combine different
physics-based observation models with data-driven image priors
in the form of denoisers, and achieve state-of-the-art image reconstruction
quality in many applications. In this paper, we aim to further
improve the computational efficacy of PnP methods by designing
a new algorithm that makes use of stochastic variance-reduced
gradients (SVRG), a nascent idea to accelerate runtime in stochastic
optimization. Compared with existing PnP methods using batch gradients
or stochastic gradients, the new algorithm, called PnP-SVRG,
achieves comparable or better accuracy of image reconstruction at a
much faster computational speed. Extensive numerical experiments
are provided to demonstrate the benefits of the proposed algorithm
through the application of compressive imaging using partial Fourier
measurements in conjunction with a wide variety of popular image
denoisers.