Documents
Poster
Poster for ICASSP 2024 paper "Hot-Fixing Wake Work Recognition for End-to-End ASR via Neural Model Reprogramming"
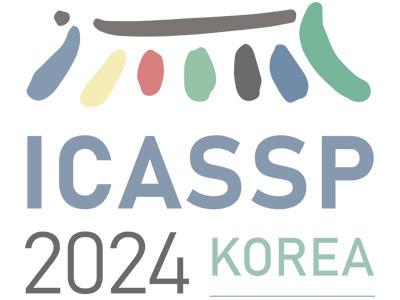
- DOI:
- 10.60864/48zd-xk03
- Citation Author(s):
- Submitted by:
- I-Fan Chen
- Last updated:
- 15 April 2024 - 10:35pm
- Document Type:
- Poster
- Document Year:
- 2024
- Event:
- Presenters:
- I-Fan Chen
- Paper Code:
- SLP-P4
- Categories:
- Keywords:
- Log in to post comments
This paper proposes two novel variants of neural reprogramming to enhance wake word recognition in streaming end-to-end ASR models without updating model weights. The first, "trigger-frame reprogramming", prepends the input speech feature sequence with the learned trigger-frames of the target wake word to adjust ASR model’s hidden states for improved wake word recognition. The second, "predictor-state initialization", trains only the initial state vectors (cell and hidden states) of the LSTMs in the prediction network. When applying to a baseline LibriSpeech Emformer RNN-T model with a 98% wake word verification false rejection rate (FRR) on unseen wake words, the proposed approaches achieve 76% and 97% relative FRR reductions with no increase on false acceptance rate. In-depth characteristic analyses of the proposed approaches are also conducted to provide deeper insights. These approaches offer an effective hot-fixing methods to improve wake word recognition performance in deployed production ASR models without the need for model updates.