Documents
Poster
PRE-TRAINING OF SPEAKER EMBEDDINGS FOR LOW-LATENCY SPEAKER CHANGE DETECTION IN BROADCAST NEWS
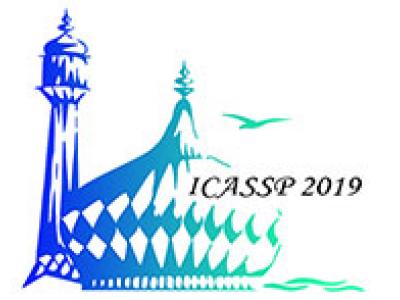
- Citation Author(s):
- Submitted by:
- LEDA SARI
- Last updated:
- 8 May 2019 - 11:35am
- Document Type:
- Poster
- Document Year:
- 2019
- Event:
- Presenters:
- Leda Sari
- Paper Code:
- 3093
- Categories:
- Log in to post comments
In this work, we investigate pre-training of neural network based speaker embeddings for low-latency speaker change detection. Our proposed system takes two speech segments, generates embeddings using shared Siamese layers and then classifies the concatenated embeddings depending on whether they are spoken by the same speaker. We investigate gender classification, contrastive loss and triplet loss based pre-training of the embedding layers and also joint training of the embedding layers along with a same/different classifier. Training is performed on 2-second single speaker segments based on ground truth speaker segmentation of broadcast news data. However, during test, we use the detection system in a practical low-latency setting for annotating automatic closed captions. In contrast to training, test pairs are now created around automatic speech recognition(ASR) based segmentation boundaries. The ASR segments are often shorter than 2 seconds causing duration mismatch during testing. In our experiments, although the baseline i-vector based classifier performs well, the proposed triplet loss based pre-training followed by joint training provides 7-50% relative F-measure improvement in matched and mismatched conditions. In addition, the degradation in performance is less severe for network based embeddings as compared to using i-vectors in the variable duration test conditions.
https://ieeexplore.ieee.org/document/8683612