Documents
Presentation Slides
Probabilistic Graph Neural Networks for Traffic Signal Control
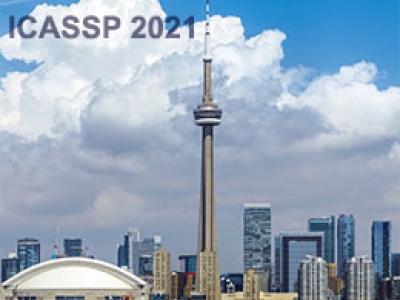
- Citation Author(s):
- Submitted by:
- Zheyang Xu
- Last updated:
- 16 June 2021 - 11:15am
- Document Type:
- Presentation Slides
- Document Year:
- 2021
- Event:
- Presenters:
- Zheyang Xu
- Paper Code:
- MLSP-47.6:
- Categories:
- Log in to post comments
Intelligent traffic signal control is crucial for efficient
transportation systems. Recent studies use reinforcement
learning (RL) to coordinate traffic signals and improve traffic
signal cooperation. However, they either design the state of
agents in a heuristic manner or model traffic dynamics in a deterministic way. This work presents a variational graph learning model TSC-GNN (Traffic Signal Control via probabilistic
Graph Neural Networks) to learn the latent representations of
agents and generate Q-value while taking traffic uncertainty
conditions into account. Besides, we explain the rationality
behind our state design using transportation theory. Experimental results conducted on real-world datasets demonstrate
our model’s superiority, e.g., it achieves more than 8% traffic efficiency improvement compared with the state-of-the-art
baselines