Documents
Presentation Slides
Progressive Continual Learning for Spoken Keyword Spotting
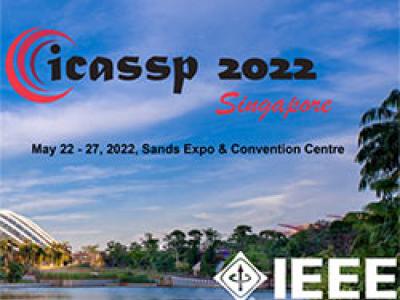
- Citation Author(s):
- Submitted by:
- Yizheng Huang
- Last updated:
- 4 May 2022 - 10:59pm
- Document Type:
- Presentation Slides
- Document Year:
- 2022
- Event:
- Presenters:
- Yizheng Huang
- Paper Code:
- 2838
- Categories:
- Keywords:
- Log in to post comments
Catastrophic forgetting is a thorny challenge when updating keyword spotting (KWS) models after deployment. To tackle such challenges, we propose a progressive continual learning strategy for small-footprint spoken keyword spotting (PCL-KWS). Specifically, the proposed PCL-KWS framework introduces a network instantiator to generate the task-specific sub-networks for remembering previously learned keywords. As a result, the PCL-KWS approach incrementally learns new keywords without forgetting prior knowledge. Besides, the keyword-aware network scaling mechanism of PCL-KWS constrains the growth of model parameters while achieving high performance. Experimental results show that after learning five new tasks sequentially, our proposed PCL-KWS approach archives the new state-of-the-art performance of 92.8% average accuracy for all the tasks on Google Speech Command dataset compared with other baselines.