Documents
Poster
Proximal Deep Recurrent Neural Network for Monaural Singing Voice Separation
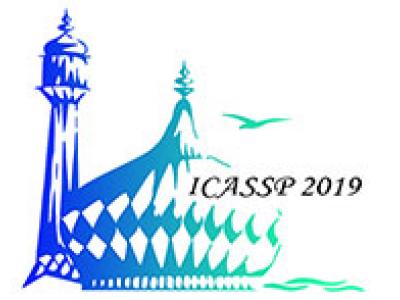
- Citation Author(s):
- Submitted by:
- Weitao Yuan
- Last updated:
- 8 May 2019 - 2:03am
- Document Type:
- Poster
- Document Year:
- 2019
- Event:
- Presenters:
- Weitao Yuan
- Paper Code:
- ICASSP19005
- Categories:
- Keywords:
- Log in to post comments
The recent deep learning methods can offer state-of-the-art performance for Monaural Singing Voice Separation (MSVS). In these deep methods, the recurrent neural network (RNN) is widely employed. This work proposes a novel type of Deep RNN (DRNN), namely Proximal DRNN (P-DRNN) for MSVS, which improves the conventional Stacked RNN (S-RNN) by introducing a novel interlayer structure. The interlayer structure is derived from an optimization problem for Monaural Source Separation (MSS). Accordingly, this enables a new hierarchical processing in the proposed P-DRNN with the explicit state transfers between different layers and the skip connections from the inputs, which are efficient for source separation. Finally, the proposed approach is evaluated on the MIR-1K dataset to verify its effectiveness. The numerical results show that the P-DRNN performs better than the conventional S-RNN and several recent MSVS methods.