Documents
Poster
Poster
Quantum Privacy Aggregation of Teacher Ensembles (QPATE) for Privacy-preserving Quantum Machine Learning
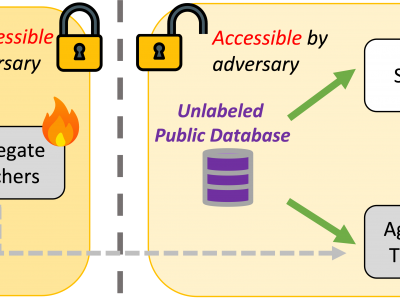
- DOI:
- 10.60864/zrqc-1n58
- Citation Author(s):
- Submitted by:
- Heehwan Wang
- Last updated:
- 6 June 2024 - 10:32am
- Document Type:
- Poster
- Document Year:
- 2024
- Event:
- Categories:
- Log in to post comments
The utility of machine learning has rapidly expanded in the last two decades and presented an ethical challenge. Papernot et. al. developed a technique, known as Private Aggregation of Teacher Ensembles (PATE) to enable federated learning in which multiple \emph{distributed teachers} are trained on disjoint data sets. This study is the first to apply PATE to an ensemble of quantum neural networks (QNN) to pave a new way of ensuring privacy in quantum machine learning (QML).