Documents
Presentation Slides
RADAR PERCEPTION WITH SCALABLE CONNECTIVE TEMPORAL RELATIONS FOR AUTONOMOUS DRIVING
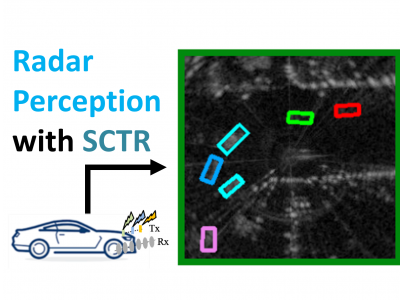
- DOI:
- 10.60864/mwf1-rc59
- Citation Author(s):
- Submitted by:
- Ryoma Yataka
- Last updated:
- 6 June 2024 - 10:21am
- Document Type:
- Presentation Slides
- Document Year:
- 2024
- Event:
- Presenters:
- Ryoma Yataka
- Paper Code:
- SS-L2.3
- Categories:
- Keywords:
- Log in to post comments
Due to the noise and low spatial resolution in automotive radar data, exploring temporal relations of learnable features over consecutive 2 radar frames has shown performance gain on downstream tasks (e.g., object detection and tracking) in our previous study. In this paper, we further enhance radar perception by significantly extending the time horizon of temporal relations.
To this end, we propose a scalable connective temporal radar (SCTR) method that consists of 1) a standard temporal relation layer (TRL), 2) a connective TRL with shifted window attention, and 3) a window merging operation, to facilitate feature connectivity between radar frames over an extended time interval. Our complexity analysis and comprehensive evaluation of the Radiate dataset demonstrate that the SCTR achieves a great tradeoff between the complexity and downstream detection performance.