Documents
Research Manuscript
Rain Estimation Over a Region Using CycleGan
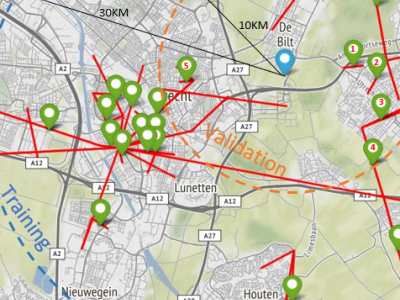
- Citation Author(s):
- Submitted by:
- Sergey Timinsky
- Last updated:
- 25 May 2023 - 6:03am
- Document Type:
- Research Manuscript
- Document Year:
- 2023
- Event:
- Presenters:
- Sergey (Sagi) Timinsky
- Categories:
- Log in to post comments
In the last couple of years, supervised machine learning (ML) methods have shown state-of-the-art results for near-ground rain estimation. Information is usually obtained from two kinds of sensors - rain gauges, which measure rain rate, and commercial microwave links (CMLs) which measure attenuation. These data sources are paired to create a dataset on which a model is trained.
The arising problem of such methods of training is in the need for the datasets to be constructed with a CML-rain gauge pairing relation.
In this paper, we propose a novel approach for rain estimation using a training method that does not require a matching between a CML and a rain gauge. Our goal is to infer the relation between CML measurements to rain rate values, with a data-driven approach using an unpaired dataset.
We achieve this by inducing two cycle-consistency losses that capture the intuition that if we translate from attenuation measurements to rain rate observations and back again - we should arrive at where we started. Moreover, we learn two mapping functions translating between A (attenuation) and R (rain-rate), denoted by G:A->R and F:R->A. No information is provided as to which sample in A matches which sample in R.
We demonstrate our results using estimated accumulated rain predictions and validate them with a nearby rain gauge station.