Documents
Presentation Slides
Recurrent Phase Reconstruction Using Estimated Phase Derivatives from Deep Neural Networks
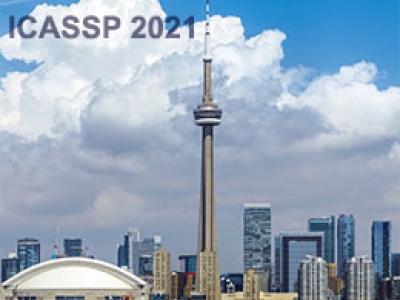
- Citation Author(s):
- Submitted by:
- Lars Thieling
- Last updated:
- 5 July 2021 - 10:03am
- Document Type:
- Presentation Slides
- Document Year:
- 2021
- Event:
- Presenters:
- Lars Thieling
- Paper Code:
- SPE-50.5
- Categories:
- Log in to post comments
This paper presents a deep neural network (DNN)-based system for phase reconstruction of speech signals solely from their magnitude spectrograms. The phase is very sensitive to time shifts. Therefore it is meaningful to estimate the phase derivatives instead of the phase directly, e.g., using DNNs and then apply a phase reconstruction method to recombine these estimates to a suitable phase spectrum. In this paper, we propose three changes for such a two-stage phase reconstruction system. For estimating phase derivatives using DNNs, we propose 1) a new regularized cosine loss function and 2) a preprocessing step for the phase derivatives that eliminates systematic offsets in the data. The experimental results demonstrate that these two changes reduce the training duration and stabilize the training with respect to hyperparameter variations. Furthermore, we propose 3) a new simple but effective averaging of weighted derivative estimates for the subsequent phase reconstruction method. Experimental results confirm that the proposed reconstruction method surpasses state-of-the-art systems both in terms of the reconstructed phase spectra and the overall achieved speech quality.