Documents
Poster
REDAT: ACCENT-INVARIANT REPRESENTATION FOR END-TO-END ASR BY DOMAIN ADVERSARIAL TRAINING WITH RELABELING
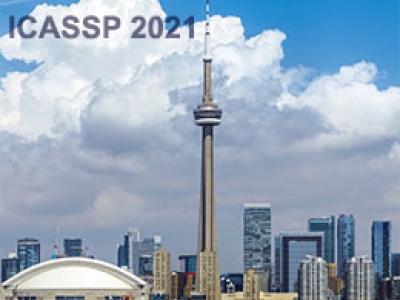
- Citation Author(s):
- Submitted by:
- Hu Hu
- Last updated:
- 21 June 2021 - 5:48pm
- Document Type:
- Poster
- Document Year:
- 2021
- Event:
- Presenters:
- Hu Hu
- Paper Code:
- 2760
- Categories:
- Log in to post comments
Accents mismatching is a critical problem for end-to-end ASR. This paper aims to address this problem by building an accent-robust RNN-T system with domain adversarial training (DAT). We unveil the magic behind DAT and provide, for the first time, a theoretical guarantee that DAT learns accent-invariant representations. We also prove that performing the gradient reversal in DAT is equivalent to minimizing the Jensen-Shannon divergence between domain output distributions. Motivated by the proof of equivalence, we introduce reDAT, a novel technique based on DAT, which relabels data using either unsupervised clustering or soft labels. Experiments on 23K hours of multi-accent data show that DAT achieves competitive results over accent-specific baselines on both native and non-native English accents but up to 13% relative WER reduction on unseen accents; our reDAT yields further improvements over DAT by 3% and 8% relatively on non-native accents of American and British English.