Documents
Presentation Slides
ROBUST DISENTANGLED VARIATIONAL SPEECH REPRESENTATION LEARNING FOR ZERO-SHOT VOICE CONVERSION
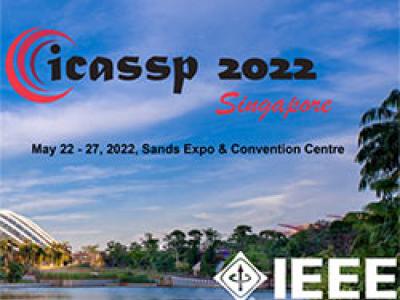
- Citation Author(s):
- Submitted by:
- Jiachen Lian
- Last updated:
- 4 May 2022 - 10:14pm
- Document Type:
- Presentation Slides
- Document Year:
- 2022
- Event:
- Presenters:
- Jiachen Lian
- Categories:
- Log in to post comments
Traditional studies on voice conversion (VC) have made progress with parallel training data and known speakers. Good voice conversion quality is obtained by exploring better alignment modules or expressive mapping functions. In this study, we investigate zero-shot VC from a novel perspective of self-supervised disentangled speech representation learning. Specifically, we achieve the disentanglement by balancing the information flow between global speaker representation and time-varying content representation in a sequential variational autoencoder (VAE). A zero-shot voice conversion is performed by feeding an arbitrary speaker embedding and content embeddings to the VAE decoder. Besides that, an on-the-fly data augmentation training strategy is applied to make the learned representation noise invariant. On TIMIT and VCTK datasets, we achieve state-of-the-art performance on both objective evaluation, i.e., speaker verification (SV) on speaker embedding and content embedding, and subjective evaluation, i.e., voice naturalness and similarity, and remains to be robust even with noisy source/target utterances.