Documents
Presentation Slides
Robust Domain-Free Domain Generalization with Class-Aware Alignment
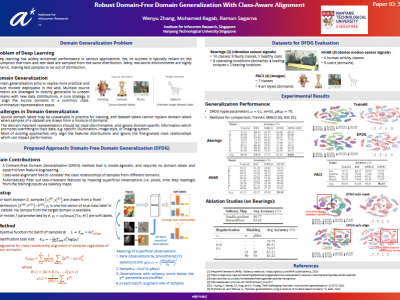
- Citation Author(s):
- Submitted by:
- Wenyu Zhang
- Last updated:
- 22 June 2021 - 1:27am
- Document Type:
- Presentation Slides
- Document Year:
- 2021
- Event:
- Presenters:
- Wenyu Zhang
- Paper Code:
- MLSP-5.6
- Categories:
- Log in to post comments
While deep neural networks demonstrate state-of-the-art performance on a variety of learning tasks, their performance relies on the assumption that train and test distributions are the same, which may not hold in real-world applications. Domain generalization addresses this issue by employing multiple source domains to build robust models that can generalize to unseen target domains subject to shifts in data distribution. In this paper, we propose Domain-Free Domain Generalization (DFDG), a model-agnostic method to achieve better generalization performance on the unseen test domain without the need for source domain labels. DFDG uses novel strategies to learn domain-invariant class-discriminative features. It aligns class relationships of samples through class-conditional soft labels, and uses saliency maps, traditionally developed for post-hoc analysis of image classification networks, to remove superficial observations from training inputs. DFDG obtains competitive performance on both time series sensor and image classification public datasets.
DFDG_slides.pdf

DFDG_poster.pdf
