Documents
Presentation Slides
Robust Self-Supervised Learning With Contrast Samples For Natural Language Understanding
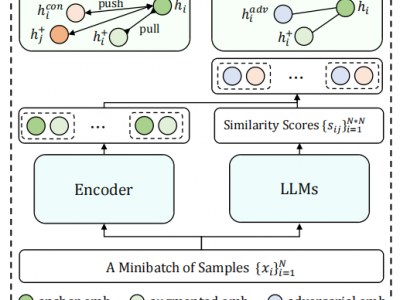
- DOI:
- 10.60864/gkwm-td51
- Citation Author(s):
- Submitted by:
- Xue Han
- Last updated:
- 6 June 2024 - 10:32am
- Document Type:
- Presentation Slides
- Event:
- Presenters:
- Xue Han
- Paper Code:
- SLP-L19.6
- Categories:
- Keywords:
- Log in to post comments
To improve the robustness of pre-trained language models (PLMs), previous studies have focused more on how to efficiently obtain adversarial samples with similar semantics, but less attention has been paid to the perturbed samples that change the gold label. Therefore, to fully perceive the effects of these different types of small perturbations on robustness, we propose a RObust Self-supervised leArning (ROSA) method, which incorporates different types of perturbed samples and the robustness improvements into a unified framework. Subsequently, to implement ROSA, a perturbed sample generation strategy supported by the large language models (LLMs) is proposed, which adaptively controls the generation process based on the fine-grained similarity information among the training samples. The experimental results demonstrate the remarkable performance of our ROSA.