Documents
Presentation Slides
Presentation Slides
SAFE SCREENING FOR SPARSE REGRESSION WITH THE KULLBACK-LEIBLER DIVERGENCE
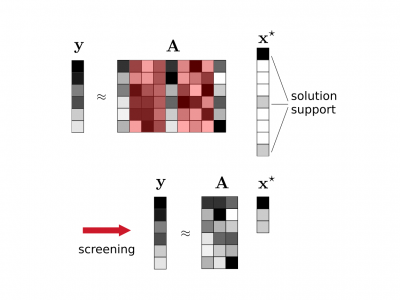
- Citation Author(s):
- Submitted by:
- Cassio FRAGA DANTAS
- Last updated:
- 27 June 2021 - 8:09am
- Document Type:
- Presentation Slides
- Document Year:
- 2021
- Event:
- Presenters:
- Cassio F. Dantas
- Paper Code:
- SPTM-21.4
- Categories:
- Log in to post comments
Safe screening rules are powerful tools to accelerate iterative solvers in sparse regression problems. They allow early identification of inactive coordinates (i.e., those not belonging to the support of the solution) which can thus be screened out in the course of iterations. In this paper, we extend the GAP Safe screening rule to the L1-regularized Kullback-Leibler divergence which does not fulfill the regularity assumptions made in previous works. The proposed approach is experimentally validated on synthetic and real count data sets.