Documents
Presentation Slides
saito22icassp_slide
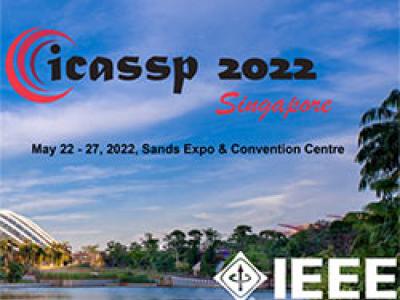
- Citation Author(s):
- Submitted by:
- Yuki Saito
- Last updated:
- 24 May 2022 - 1:30pm
- Document Type:
- Presentation Slides
- Document Year:
- 2022
- Event:
- Presenters:
- Yuki Saito
- Paper Code:
- SPE-L3.9
- Categories:
- Log in to post comments
We propose novel deep speaker representation learning that considers perceptual similarity among speakers for multi-speaker generative modeling. Following its success in accurate discriminative modeling of speaker individuality, knowledge of deep speaker representation learning (i.e., speaker representation learning using deep neural networks) has been introduced to multi-speaker generative modeling. However, the conventional discriminative algorithm does not necessarily learn speaker embeddings suitable for such generative modeling, which may result in lower quality and less controllability of synthetic speech. We propose three representation learning algorithms that utilize a perceptual speaker similarity matrix obtained by large-scale perceptual scoring of speaker-pair similarity. The algorithms train a speaker encoder to learn speaker embeddings with three different representations of the matrix: a set of vectors, the Gram matrix, and a graph. Furthermore, we propose an active learning algorithm that iterates the perceptual scoring and speaker encoder training. To obtain accurate embeddings while reducing costs of scoring and training, the algorithm selects unscored speaker-pairs to be scored next on the basis of the sequentially-trained speaker encoder's similarity prediction results. Experimental evaluation results show that 1) the proposed representation learning algorithms learn speaker embeddings strongly correlated with perceptual speaker-pair similarity, 2) the embeddings improve synthetic speech quality in speech autoencoding tasks better than conventional d-vectors learned by discriminative modeling, 3) the proposed active learning algorithm achieves higher synthetic speech quality while reducing costs of scoring and training, and 4) among the proposed similarity {vector, matrix, graph} embedding algorithms, the first achieves the best speaker similarity for synthetic speech and the third gives the most improvement in the synthetic speech naturalness.