Documents
Presentation Slides
Semantic Image Segmentation Guided by Scene Geometry
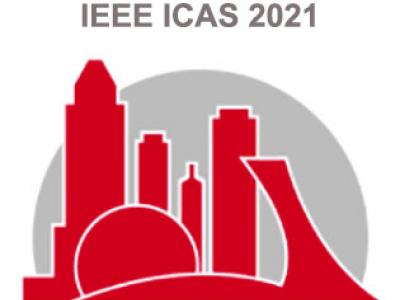
- Citation Author(s):
- Submitted by:
- Sotirios Papado...
- Last updated:
- 25 August 2021 - 5:49am
- Document Type:
- Presentation Slides
- Document Year:
- 2021
- Event:
- Presenters:
- Ioannis Pitas
- Categories:
- Log in to post comments
Semantic image segmentation is an important functionality in various applications, such as robotic vision for autonomous cars, drones, etc. Modern Convolutional Neural Networks (CNNs) process input RGB images and predict per-pixel semantic classes. Depth maps have been successfully utilized to increase accuracy over RGB-only input. They can be used as an additional input channel complementing the RGB image, or they may be estimated by an extra neural branch under a multitask training setting. Contrary to these approaches, in this paper we explore a novel regularizer that penalizes differences between semantic and self-supervised depth predictions on presumed object boundaries during CNN training. The proposed method does not resort to multitask training (which may require a more complex CNN backbone to avoid underfitting), does not rely on RGB-D or stereoscopic 3D training data and does not require known or estimated depth maps during inference. Quantitative evaluation on a public scene parsing video dataset for autonomous driving indicates enhanced semantic segmentation accuracy with zero inference runtime overhead.