Documents
Poster
Poster
SEMI-SUPERVISED GRAPHICAL DEEP DICTIONARY LEARNING FOR HYPERSPECTRAL IMAGE CLASSIFICATION FROM LIMITED SAMPLES
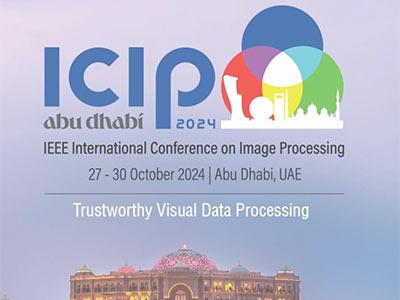
- Citation Author(s):
- Submitted by:
- Anurag Goel
- Last updated:
- 8 November 2024 - 10:14am
- Document Type:
- Poster
- Document Year:
- 2024
- Event:
- Presenters:
- Anurag Goel
- Paper Code:
- 1202
- Categories:
- Keywords:
- Log in to post comments
In this work, we propose a semi-supervised deep feature generation network that accounts for local similarities. It is based on the deep dictionary learning (DDL) framework. The formulation accounts for two unique aspects of hyperspectral classification. First, the fact that the total number of pixels / samples to be labeled is constant; this allows for a semi-supervised formulation allowing only a few pixels / samples to be labeled as training data. Second, the samples / pixels are spatially correlated; this leads to a graph regularization formulation. Our formulation has been benchmarked with state-of-the-art techniques on two popular datasets; the results show that our work improves upon the ones compared against.