Documents
Presentation Slides
SEMI-SUPERVISED TRAINING OF ACOUSTIC MODELS USING LATTICE-FREE MMI
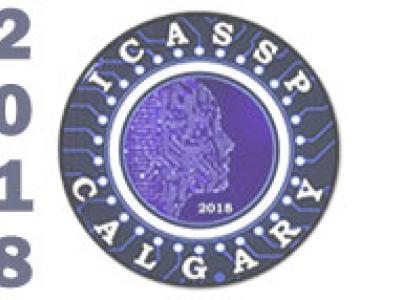
- Citation Author(s):
- Submitted by:
- Vimal Manohar
- Last updated:
- 18 April 2018 - 12:19pm
- Document Type:
- Presentation Slides
- Document Year:
- 2018
- Event:
- Presenters:
- Vimal Manohar
- Paper Code:
- SP-L4.2
- Categories:
- Keywords:
- Log in to post comments
The lattice-free MMI objective (LF-MMI) has been used in supervised training of
state-of-the-art neural network acoustic models for automatic speech
recognition (ASR). With large amounts of unsupervised data available,
extending this approach to the semi-supervised scenario is of significance.
Finite-state transducer (FST) based supervision used with LF-MMI provides a
natural way to incorporate uncertainties when dealing with unsupervised data.
In this paper,
we describe various extensions to standard LF-MMI training to allow the use
as supervision of lattices obtained via decoding of unsupervised data. The
lattices are rescored with a strong LM. We
investigate different methods for splitting the lattices and incorporating
frame tolerances into the supervision FST. We report results on
different subsets of Fisher English, where we achieve WER recovery of
59-64\% using lattice supervision, which is significantly better than
using just the best path transcription.