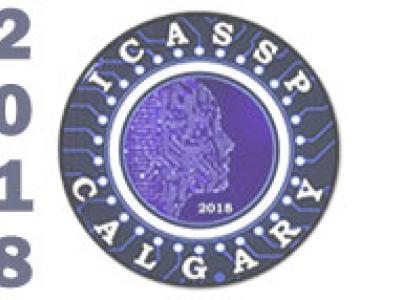
- Read more about SEMI-SUPERVISED TRAINING OF ACOUSTIC MODELS USING LATTICE-FREE MMI
- Log in to post comments
The lattice-free MMI objective (LF-MMI) has been used in supervised training of
state-of-the-art neural network acoustic models for automatic speech
recognition (ASR). With large amounts of unsupervised data available,
extending this approach to the semi-supervised scenario is of significance.
Finite-state transducer (FST) based supervision used with LF-MMI provides a
natural way to incorporate uncertainties when dealing with unsupervised data.
In this paper,
we describe various extensions to standard LF-MMI training to allow the use
- Categories: