Documents
Poster
SEQUENTIAL STRUCTURED DICTIONARY LEARNING FOR BLOCK SPARSE REPRESENTATIONS
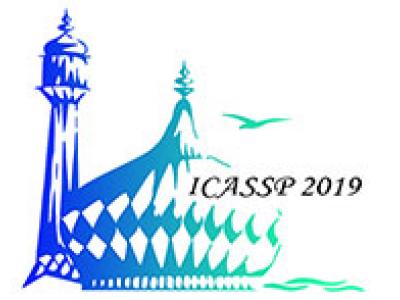
- Citation Author(s):
- Submitted by:
- Asif Iqbal
- Last updated:
- 10 May 2019 - 1:47am
- Document Type:
- Poster
- Document Year:
- 2019
- Event:
- Presenters:
- Abd-Krim Seghouane
- Paper Code:
- 2601
- Categories:
- Log in to post comments
Dictionary learning algorithms have been successfully applied to a number of signal and image processing problems. In some applications however, the observed signals may have a multi-subpsace structure that enables block-sparse signal representations. Based on the observation that the observed signals can be approximated as a sum of low rank matrices, a new algorithm for learning a block-structured dictionary for block-sparse signal representations is proposed. It’s derived via sequential penalized low rank matrix approximation, where a block coordinate descent approach is used to estimate the matrix pairs that form the different low rank matrix approximations. Experimental results on synthetic and standard gray-scale images illustrating the superior performance of the proposed algorithm are provided.