Documents
Research Manuscript
SPASE: SPAtial Saliency Explanation for time series models
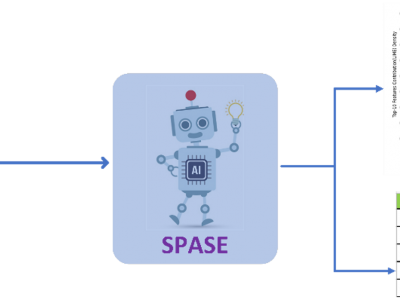
- DOI:
- 10.60864/vxmx-yr55
- Citation Author(s):
- Submitted by:
- PRANAY LOHIA
- Last updated:
- 29 March 2024 - 3:45am
- Document Type:
- Research Manuscript
- Document Year:
- 2024
- Event:
- Presenters:
- Siddarth Asokan
- Paper Code:
- MLSP-L17.3
- Categories:
- Log in to post comments
We have seen recent advances in the fields of Machine Learning (ML), Deep Learning (DL), and Artificial intelligence (AI) that the models are becoming increasingly complex and large in terms of architecture and parameter size. These complex ML/DL models have beaten the state of the art in most fields of computer science like computer vision, NLP, tabular data prediction and time series forecasting, etc. With the increase in models’ performance, model explainability and interpretability has become essential to explain/justify model outcome, especially for business use cases. There has been significant improvement in the domain of model explainability for Computer Vision and Natural Language Processing (NLP) tasks with fundamental research for both black-box and white-box techniques. In this paper, we proposed novel time series explainability techniques SPASE for black-box time series model forecasting and anomaly detection problems.
Comments
Documents uploaded
Paper pre-print submitted