Documents
Poster
SPE-89.4: UNSUPERVISED DATA SELECTION FOR SPEECH RECOGNITION WITH CONTRASTIVE LOSS RATIOS
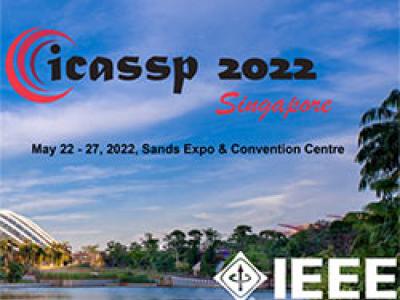
- Citation Author(s):
- Submitted by:
- Chanho Park
- Last updated:
- 26 May 2022 - 5:50am
- Document Type:
- Poster
- Document Year:
- 2022
- Event:
- Presenters:
- Chanho Park
- Paper Code:
- SPE-89.4
- Categories:
- Log in to post comments
This paper proposes an unsupervised data selection method by using a submodular function based on contrastive loss ratios of target and training data sets. A model using a contrastive loss function is trained on both sets. Then the ratio of frame-level losses for each model is used by a submodular function. By using the submodular function, a training set for automatic speech recognition matching the target data set is selected. Experiments show that models trained on the data sets selected by the proposed method outperform the selection method based on log-likelihoods produced by GMM-HMM models, in terms of word error rate (WER). When selecting a fixed amount, e.g. 10 hours of data, the difference between the results of two methods on Tedtalks was 20.23% WER relative. The method can also be used to select data with the aim of minimising negative transfer, while maintaining or improving on performance of models trained on the whole training set. Results show that the WER on the WSJCAM0 data set was reduced by 6.26% relative when selecting 85% from the whole data set.