Documents
Poster
Speaker-Invariant Training via Adversarial Learning
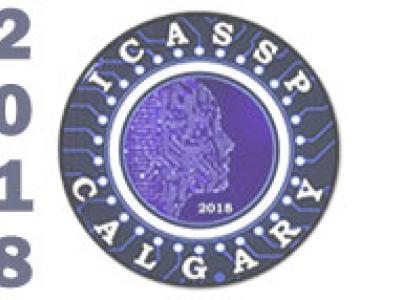
- Citation Author(s):
- Submitted by:
- Zhong Meng
- Last updated:
- 12 May 2019 - 9:29pm
- Document Type:
- Poster
- Document Year:
- 2018
- Event:
- Presenters:
- Yifan Gong
- Paper Code:
- 2213
- Categories:
- Log in to post comments
We propose a novel adversarial multi-task learning scheme, aiming at actively curtailing the inter-talker feature variability while maximizing its senone discriminability so as to enhance the performance of a deep neural network (DNN) based ASR system. We call the scheme speaker-invariant training (SIT). In SIT, a DNN acoustic model and a speaker classifier network are jointly optimized to minimize the senone (tied triphone state) classification loss, and simultaneously mini-maximize the speaker classification loss. A speaker-invariant and senone-discriminative deep feature is learned through this adversarial multi-task learning. With SIT, a canonical DNN acoustic model with significantly reduced variance in its output probabilities is learned with no explicit speaker-independent (SI) transformations or speaker-specific representations used in training or testing. Evaluated on the CHiME-3 dataset, the SIT achieves 4.99% relative word error rate (WER) improvement over the conventional SI acoustic model. With additional unsupervised speaker adaptation, the speaker-adapted (SA) SIT model achieves 4.86% relative WER gain over the SA SI acoustic model.