Documents
Poster
SPECTRAL DISTORTION MODEL FOR TRAINING PHASE-SENSITIVE DEEP-NEURAL NETWORKS FOR FAR-FIELD SPEECH RECOGNITION
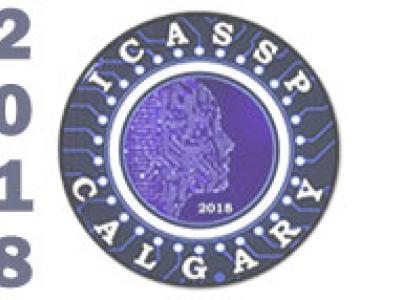
- Citation Author(s):
- Submitted by:
- Chanwoo Kim
- Last updated:
- 7 May 2018 - 12:19am
- Document Type:
- Poster
- Document Year:
- 2018
- Event:
- Presenters:
- Chanwoo Kim
- Paper Code:
- ICASSP18001 4
- Categories:
- Log in to post comments
In this paper, we present an algorithm which introduces phase-perturbation to the training database when training phase-sensitive deep neural-network models. Traditional features such as log-mel or cepstral features do not have have any phase-relevant information.However features such as raw-waveform or complex spectra features contain phase-relevant information. Phase-sensitive features have the advantage of being able to detect differences in time of
arrival across different microphone channels or frequency bands. However, compared to magnitude-based features, phase information is more sensitive to various kinds of distortions such as variations in microphone characteristics, reverberation, and so on. For traditional magnitude-based features, it is widely known that adding noise or reverberation, often called Multistyle-TRaining (MTR), improves robustness. In a similar spirit, we propose an algorithm which introduces spectral distortion to make the deep-learning models more robust to phase-distortion. We call this approach Spectral-Distortion TRaining (SDTR). In our experiments using a training set consisting of 22-million utterances with and without MTR, this approach reduces Word Error Rates (WERs) relatively by 3.2 % and 8.48 % respectively on test sets recorded on Google Home.