Documents
Poster
Speech Dereverberation based on Convex Optimization Algorithms for Group Sparse Linear Prediction
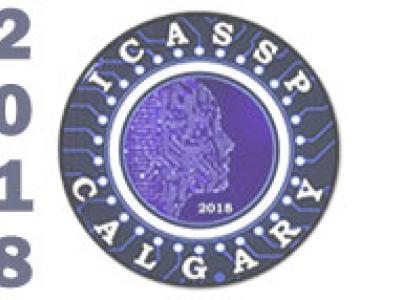
- Citation Author(s):
- Submitted by:
- Daniele Giacobello
- Last updated:
- 12 April 2018 - 4:04pm
- Document Type:
- Poster
- Document Year:
- 2018
- Event:
- Paper Code:
- AASP-P5
- Categories:
- Log in to post comments
In this paper, we consider methods for improving far-field speech recognition using dereverberation based on sparse multi-channel linear prediction. In particular, we extend successful methods based on nonconvex iteratively reweighted least squares, that look for a sparse desired speech signal in the short-term Fourier transform domain, by proposing sparsity promoting convex functions. Furthermore, we show how to improve performance by applying regularization into both the reweighted least squares and convex methods. We evaluate the methods using large scale simulations by mimicking the application scenarios of interest. The experiments show that the proposed convex formulations and regularization offer improvements over existing methods with added robustness and flexibility in fairly different acoustic scenarios.