Documents
Presentation Slides
Speech Landmark Bigrams for Depression Detection from Naturalistic Smartphone Speech
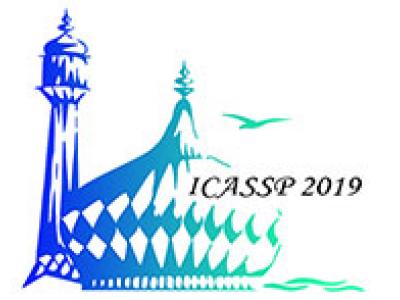
- Citation Author(s):
- Submitted by:
- Zhaocheng Huang
- Last updated:
- 6 June 2019 - 4:42am
- Document Type:
- Presentation Slides
- Document Year:
- 2019
- Event:
- Presenters:
- Zhaocheng Huang
- Paper Code:
- 2788
- Categories:
- Log in to post comments
Detection of depression from speech has attracted significant research attention in recent years but remains a challenge, particularly for speech from diverse smartphones in natural environments. This paper proposes two sets of novel features based on speech landmark bigrams associated with abrupt speech articulatory events for depression detection from smartphone audio recordings. Combined with techniques adapted from natural language text processing, the proposed features further exploit landmark bigrams by discovering latent articulatory events. Experimental results on a large, naturalistic corpus containing various spoken tasks recorded from diverse smartphones suggest that speech landmark bigram features provide a 30.1% relative improvement in F1 (depressed) relative to an acoustic feature baseline system. As might be expected, a key finding was the importance of tailoring the choice of landmark bigrams to each elicitation task, revealing that different aspects of speech articulation are elicited by different tasks, which can be effectively captured by the landmark approaches.