Documents
Poster
Poster
SPIKING STRUCTURED STATE SPACE MODEL FOR MONAURAL SPEECH ENHANCEMENT
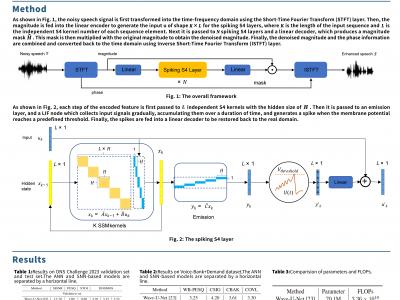
- Citation Author(s):
- Submitted by:
- Xu Liu
- Last updated:
- 15 April 2024 - 4:04am
- Document Type:
- Poster
- Document Year:
- 2024
- Event:
- Presenters:
- Xu Liu
- Categories:
- Log in to post comments
Speech enhancement seeks to extract clean speech from noisy signals. Traditional deep learning methods face two challenges: efficiently using information in long speech sequences and high computational costs. To address these, we introduce the Spiking Structured State Space Model (Spiking-S4). This approach merges the energy efficiency of Spiking Neural Networks (SNN) with the long-range sequence modeling capabilities of Structured State Space Models (S4), offering a compelling solution. Evaluation on the DNS Challenge and VoiceBank+Demand Datasets confirms that Spiking-S4 rivals existing Artificial Neural Network (ANN) methods but with fewer computational resources, as evidenced by reduced parameters and Floating Point Operations (FLOPs).