Documents
Poster
Poster
Stage-Regularized Neural Stein Critics for Testing Goodness-of-Fit of Generative Models
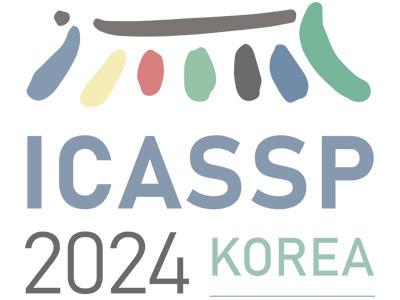
- DOI:
- 10.60864/24wj-hj52
- Citation Author(s):
- Submitted by:
- Matthew Repasky
- Last updated:
- 6 June 2024 - 10:50am
- Document Type:
- Poster
- Document Year:
- 2024
- Event:
- Presenters:
- Yao Xie
- Paper Code:
- MLSP-P1.11
- Categories:
- Log in to post comments
Learning to differentiate model distributions from observed data is a fundamental problem in statistics and machine learning, and high-dimensional data remains a challenging setting for such problems. Metrics that quantify the disparity in probability distributions, such as the Stein discrepancy, play an important role in high-dimensional statistical testing. This paper presents a method based on neural network Stein critics to distinguish between data sampled from an unknown probability distribution and a nominal model distribution with a novel staging of the weight of regularization. The benefit of using staged L2 regularization in training such critics is demonstrated on evaluating generative models of image data.