Documents
Poster
Stethoscope-Guided Supervised Contrastive Learning for Cross-domain Adaptation on Respiratory Sound Classification
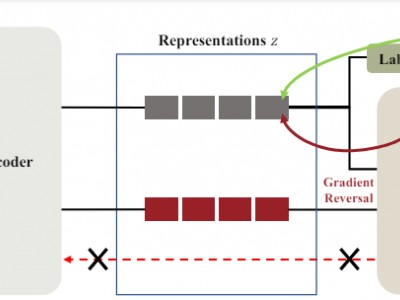
- DOI:
- 10.60864/tg1s-q455
- Citation Author(s):
- Submitted by:
- June-Woo Kim
- Last updated:
- 6 June 2024 - 10:54am
- Document Type:
- Poster
- Document Year:
- 2024
- Event:
- Presenters:
- June-Woo Kim
- Paper Code:
- AASP-P15
- Categories:
- Log in to post comments
Despite the remarkable advances in deep learning technology, achieving satisfactory performance in lung sound classification remains a challenge due to the scarcity of available data. Moreover, the respiratory sound samples are collected from a variety of electronic stethoscopes, which could potentially introduce biases into the trained models. When a significant distribution shift occurs within the test dataset or in a practical scenario, it can substantially decrease the performance. To tackle this issue, we introduce cross-domain adaptation techniques, which transfer the knowledge from a source domain to a distinct target domain. In particular, by considering different stethoscope types as individual domains, we propose a novel stethoscope-guided supervised contrastive learning approach. This method can mitigate any domain-related disparities and thus enables the model to distinguish respiratory sounds of the recording variation of the stethoscope. The experimental results on the ICBHI dataset demonstrate that the proposed methods are effective in reducing the domain dependency and achieving the ICBHI Score of 61.71%, which is a significant improvement of 2.16% over the baseline.