Documents
Presentation Slides
STRUCTURED SUPPORT EXPLORATION FOR MULTILAYER SPARSE MATRIX FACTORIZATION
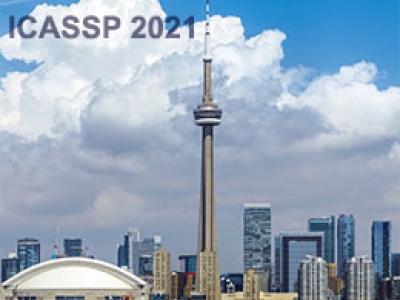
- Citation Author(s):
- Submitted by:
- TUNG LE
- Last updated:
- 25 June 2021 - 5:00am
- Document Type:
- Presentation Slides
- Document Year:
- 2021
- Event:
- Presenters:
- LE QUOC TUNG
- Paper Code:
- MLSP-18.3
- Categories:
- Log in to post comments
Matrix factorization with sparsity constraints plays an important role in many machine learning and signal processing problems such as dictionary learning, data visualization, dimension reduction. Among the most popular tools for sparse matrix factorization are proximal algorithms, a family of algorithms based on proximal operators. In this paper, we address two problems with the application of proximal algorithms to sparse matrix factorization. On the one hand, we analyze a weakness of proximal algorithms in sparse matrix factorization: the premature convergence of the support. A remedy is also proposed to address this problem. On the other hand, we describe a new tractable proximal operator called Generalized Hungarian Method, associated to so-called k-regular matrices, which are useful for the factorization of a class of matrices associated to fast linear transforms. We further illustrate the effectiveness of our proposals by numerical experiments on the Hadamard Transform and magnetoencephalography matrix factorization.