Documents
Poster
On the Supermodularity of Active Graph-based Semi-supervised Learning with Stieltjes Matrix Regularization
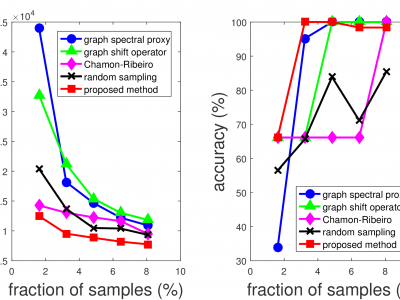
- Citation Author(s):
- Submitted by:
- Pin-Yu Chen
- Last updated:
- 20 April 2018 - 12:31am
- Document Type:
- Poster
- Document Year:
- 2018
- Event:
- Presenters:
- Pin-Yu Chen
- Paper Code:
- 3456
- Categories:
- Log in to post comments
Active graph-based semi-supervised learning (AG-SSL) aims to select a small set of labeled examples and utilize their graph-based relation to other unlabeled examples to aid in machine learning tasks. It is also closely related to the sampling theory in graph signal processing. In this paper, we revisit the original formulation of graph-based SSL and prove the supermodularity of an AG-SSL objective function under a broad class of regularization functions parameterized by Stieltjes matrices. Under this setting, supermodularity yields a novel greedy label sampling algorithm with guaranteed performance relative to the optimal sampling set. Compared to three state-of-the-art graph signal sampling and recovery methods on two real-life community detection datasets, the proposed AG-SSL method attains superior classification accuracy given limited sample budgets.