Documents
Presentation Slides
Suremap: Predicting Uncertainty in Cnn-Based Image Reconstructions Using Stein’s Unbiased Risk Estimate
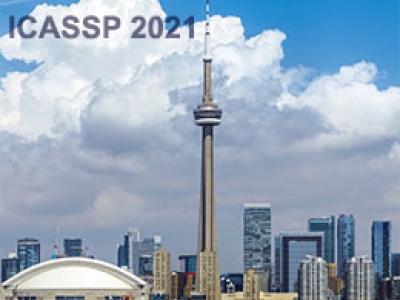
- Citation Author(s):
- Submitted by:
- Ruangrawee Kiti...
- Last updated:
- 22 June 2021 - 9:22am
- Document Type:
- Presentation Slides
- Document Year:
- 2021
- Event:
- Presenters:
- Ruangrawee Kitichotkul
- Categories:
- Log in to post comments
Convolutional neural networks (CNN) have emerged as a powerful tool for solving computational imaging reconstruction problems. However, CNNs are generally difficult-to-understand black-boxes. Accordingly, it is challenging to know when they will work and, more importantly, when they will fail. This limitation is a major barrier to their use in safety-critical applications like medical imaging: Is that blob in the reconstruction an artifact or a tumor?In this work we use Stein’s unbiased risk estimate (SURE) to develop per-pixel confidence intervals, in the form of heatmaps, for compressive sensing reconstruction using the approximate message passing (AMP) framework with CNN-based denoisers. These heatmaps tell end-users how much to trust an image formed by a CNN, which could greatly improve the utility of CNNs in various computational imaging applications.