Documents
Poster
TEMPORAL TRANSFORMER ENCODER FOR VIDEO CLASS INCREMENTAL LEARNING
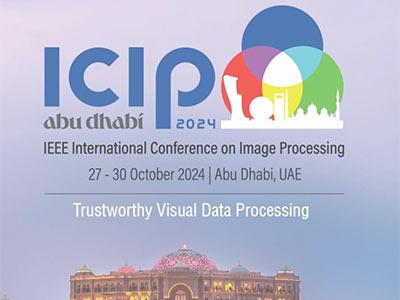
- Citation Author(s):
- Submitted by:
- Nattapong Kurpukdee
- Last updated:
- 12 November 2024 - 1:35pm
- Document Type:
- Poster
- Document Year:
- 2024
- Event:
- Presenters:
- Nattapong Kurpukdee
- Paper Code:
- TA2.PA.5
- Categories:
- Log in to post comments
Current video classification approaches suffer from catastrophic forgetting when they are retrained on new databases.
Continual learning aims to enable a classification system with learning from a succession of tasks without forgetting.
In this paper we propose to use a transformer-based video class incremental learning model. During a succession of
learning steps, at each training time, the transformer is used to extract characteristic spatio-temporal features from videos
corresponding to a set of classes. When new video classification tasks become available, we train new classifier modules
with the transformer-extracted features, gradually building a mixture model. The proposed methodology enables continual
class learning in videos without being required to consider the learning of an initial set of classes, leading to low computation and memory requirements.
The proposed model is evaluated on standard action recognition datasets including UCF101 and HMDB51, which are split into sets of classes, to be learnt sequentially.
Our proposed method significantly outperforms the baselines on all datasets.