Documents
Poster
Truncated Weighted Nuclear Norm Regularization and Sparsity for Image Denoising
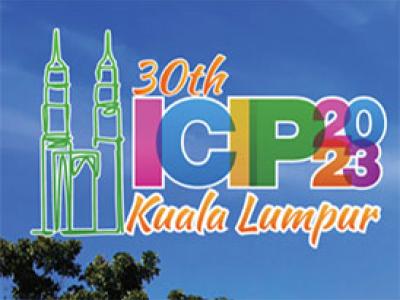
- DOI:
- 10.60864/3rrp-p639
- Citation Author(s):
- Submitted by:
- zhang mingli
- Last updated:
- 17 November 2023 - 12:05pm
- Document Type:
- Poster
- Document Year:
- 2023
- Event:
- Presenters:
- Mingli Zhang
- Categories:
- Log in to post comments
The attribute of signal sparsity is widely used to sparse representaion.
The existing nuclear norm minimization and weighted
nuclear norm minimization may achieve a suboptimal in
real application with the inaccurate approximation of rank
function. This paper presents a novel denoising method that
preserves fine structures in the image by imposing L1 norm
constraints on the wavelet transform coefficients and low
rank on high-frequency components of group similar patches.
An efficient proximal operator of Truncated Weighted Nuclear
Norm (TWNN) is proposed to accurately recover the
underlying high-frequency components of low rank patches.
By combining a wavelet domain sparse preservation prior
with TWNN, the proposed method significantly improves
the reconstruction accuracy, leading to a higher PSNR/SSIM
and visual quality than state of the art approaches.