Documents
Poster
UNRAVELING EXPLAINABLE REINFORCEMENT LEARNING USING BEHAVIOR TREE STRUCTURES
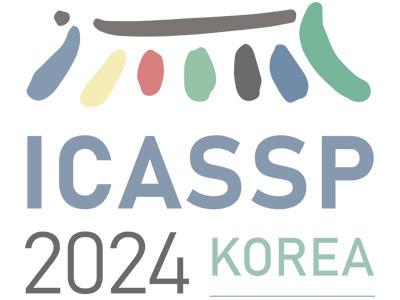
- Citation Author(s):
- Submitted by:
- Kejia Wan
- Last updated:
- 1 April 2024 - 11:00am
- Document Type:
- Poster
- Document Year:
- 2024
- Event:
- Presenters:
- Kejia Wan
- Paper Code:
- MLSP-P12.13
- Categories:
- Log in to post comments
The black-box characteristic of deep reinforcement learning restricts the safe and scalable application of decision models in practical deployment. Existing interpretability methods for deep reinforcement learning models are often inadequate in providing comprehensive insights and generating logical sequential decisions.
In this study, we propose an innovative framework called XRLBT, which introduces the behavior tree structure to explainable reinforcement learning.
XRLBT clusters state space by aggregating temporally related states. Based on these clustered states, XRLBT constructs behavior tree structures that align with the target deep reinforcement learning model.
In this way, XRLBT incorporates an exploration technique specifically designed for temporal policies in DRL.
Through extensive experimentation across six benchmark environments, we showcase the superiority of the discovered behavior tree structures over state-of-the-art algorithms.
This work represents a significant stride towards addressing the challenges of explainability and performance in DRL applications.