Documents
Poster
UNSUPERVISED LEARNING OF NEURAL SEMANTIC MAPPINGS WITH THE HUNGARIAN ALGORITHM FOR COMPOSITIONAL SEMANTICS
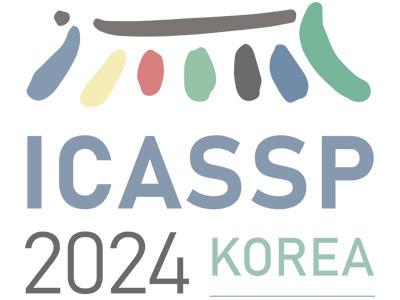
- Citation Author(s):
- Submitted by:
- Xiang Zhang
- Last updated:
- 8 April 2024 - 1:42am
- Document Type:
- Poster
- Document Year:
- 2024
- Event:
- Categories:
- Keywords:
- Log in to post comments
Neural semantic parsing maps natural languages (NL) to equivalent formal semantics which are compositional and deduce the sentence meanings by composing smaller parts. To learn a well-defined semantics, semantic parsers must recognize small parts, which are semantic mappings between NL and semantic tokens. Attentions in recent neural models are usually explained as one-on-one semantic mappings. However, attention weights with end-to-end training are shown only weakly correlated with human-labeled mappings. Despite the usefulness, supervised mappings are expensive. We propose the unsupervised Hungarian tweaks on attentions to better model mappings. Experiments have shown our methods is competitive with the supervised approach on performance and mappings recognition, and outperform other baselines.
poster.pdf
