Documents
Poster
On the Usefulness of Statistical Normalisation of Bottleneck Features for Speech Recognition
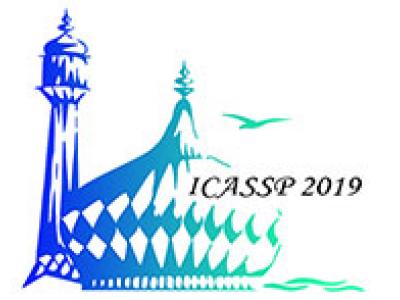
- Citation Author(s):
- Submitted by:
- Erfan Loweimi
- Last updated:
- 7 May 2019 - 1:08pm
- Document Type:
- Poster
- Document Year:
- 2019
- Event:
- Presenters:
- Erfan Loweimi
- Paper Code:
- 2446
- Categories:
- Keywords:
- Log in to post comments
DNNs play a major role in the state-of-the-art ASR systems. They can be used for extracting features and building probabilistic models for acoustic and language modelling. Despite their huge practical success, the level of theoretical understanding has remained shallow. This paper investigates DNNs from a statistical standpoint. In particular, the effect of activation functions on the distribution of the pre-activations and activations is investigated and discussed from both analytic and empirical viewpoints. This study, among others, shows that the pre-activation density in the bottleneck layer can be well fitted with a diagonal GMM with a few Gaussians and how and why the ReLU activation function promotes sparsity. Motivated by the statistical properties of the pre-activations, the usefulness of statistical normalisation of bottleneck features was also investigated. To this end, methods such as mean(-variance) normalisation, Gaussianisation, and histogram equalisation (HEQ) were employed and up to 2\% (absolute) WER reduction achieved in the Aurora-4 task.