Documents
Presentation Slides
UTILIZING SUPER-RESOLUTION FOR ENHANCED AUTOMOTIVE RADAR OBJECT DETECTION
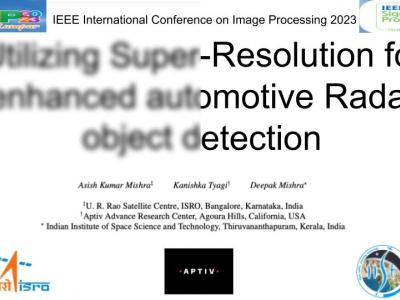
- DOI:
- 10.60864/gab6-jt61
- Citation Author(s):
- Submitted by:
- Kanishka Tyagi
- Last updated:
- 17 November 2023 - 12:05pm
- Document Type:
- Presentation Slides
- Document Year:
- 2023
- Event:
- Presenters:
- Asish Kumar Mishra
- Paper Code:
- 3444
- Categories:
- Keywords:
- Log in to post comments
In recent years, automotive radar has become an integral part of the advanced safety sensor stack. Although radar gives a significant advantage over a camera or Lidar, it suffers from poor angular resolution, unwanted noises and significant object smearing across the angular bins, making radar-based object detection challenging. We propose a novel radar-based object detection utilizing a deep learning-based super-resolution (DLSR) model. Due to the unavailability of low-high resolution radar data pair, we first simulate the data to train a DLSR model. We develop a framework that feeds a low-resolution radar dataset (called CRUW dataset) into the trained DLSR model pipeline to train a radar-based deep object detection classifier. The proposed framework achieves an 80% accuracy on object classification for the CRUW dataset and has a lower computational footprint, making it an ideal candidate for real-time implementation on edge devices used in autonomous driving applications. Code, dataset and supplementary material are on https://github.com/kanishkaisreal/DLSR_CRUW