Documents
Poster
VADOI: VOICE-ACTIVITY-DETECTION OVERLAPPING INFERENCE FOR END-TO-END LONG-FORM SPEECH RECOGNITION
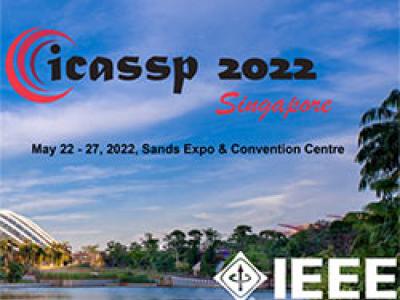
- Citation Author(s):
- Submitted by:
- Jinhan Wang
- Last updated:
- 14 May 2022 - 9:12pm
- Document Type:
- Poster
- Document Year:
- 2022
- Event:
- Presenters:
- Jinhan Wang
- Paper Code:
- SPE-34.2
- Categories:
- Log in to post comments
While end-to-end models have shown great success on the Automatic Speech Recognition task, performance degrades severely when target sentences are long-form. The previous proposed methods, (partial) overlapping inference are shown to be effective on long-form decoding. For both methods, word error rate (WER) decreases monotonically when over- lapping percentage decreases. Setting aside computational cost, the setup with 50% overlapping during inference can achieve the best performance. However, a lower overlapping percentage has an advantage of fast inference speed. In this paper, we first conduct comprehensive experiments compar- ing overlapping inference and partial overlapping inference with various configurations. We then propose Voice-Activity- Detection Overlapping Inference to provide a trade-off be- tween WER and computation cost. Results show that the pro- posed method can achieve a 20% relative computation cost reduction on Librispeech and Microsoft Speech Language Translation long-form corpus while maintaining the WER performance when comparing to the best performing over- lapping inference algorithm. We also propose Soft-Match to compensate for similar words mis-aligned problem.
VADOI Poster.pdf
