Documents
Presentation Slides
Wave-U-Net Discriminator: Fast and Lightweight Discriminator for Generative Adversarial Network-Based Speech Synthesis
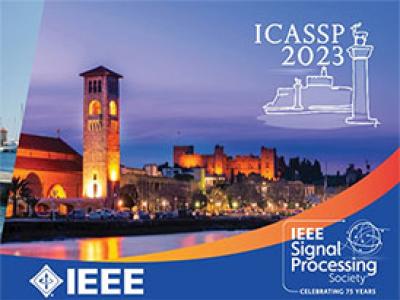
- DOI:
- 10.60864/z6xh-cw80
- Citation Author(s):
- Submitted by:
- Takuhiro Kaneko
- Last updated:
- 17 November 2023 - 12:08pm
- Document Type:
- Presentation Slides
- Document Year:
- 2023
- Event:
- Presenters:
- Takuhiro Kaneko
- Paper Code:
- SLT-L21.03
- Categories:
- Log in to post comments
In speech synthesis, a generative adversarial network (GAN), training a generator (speech synthesizer) and a discriminator in a min-max game, is widely used to improve speech quality. An ensemble of discriminators is commonly used in recent neural vocoders (e.g., HiFi-GAN) and end-to-end text-to-speech (TTS) systems (e.g., VITS) to scrutinize waveforms from multiple perspectives. Such discriminators allow synthesized speech to adequately approach real speech; however, they require an increase in the model size and computation time according to the increase in the number of discriminators. Alternatively, this study proposes a Wave-U-Net discriminator, which is a single but expressive discriminator with Wave-U-Net architecture. This discriminator is unique; it can assess a waveform in a sample-wise manner with the same resolution as the input signal, while extracting multilevel features via an encoder and decoder with skip connections. This architecture provides a generator with sufficiently rich information for the synthesized speech to be closely matched to the real speech. During the experiments, the proposed ideas were applied to a representative neural vocoder (HiFi-GAN) and an end-to-end TTS system (VITS). The results demonstrate that the proposed models can achieve comparable speech quality with a 2.31 times faster and 14.5 times more lightweight discriminator when used in HiFi-GAN and a 1.90 times faster and 9.62 times more lightweight discriminator when used in VITS. Audio samples are available at https://www.kecl.ntt.co.jp/people/kaneko.takuhiro/projects/waveunetd/.