Documents
Poster
WFTNet: Exploiting Global and Local Periodicity in Long-term Time Series Forecasting
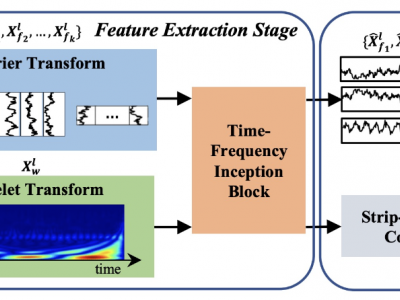
- DOI:
- 10.60864/yc4q-6s72
- Citation Author(s):
- Submitted by:
- Peiyuan Liu
- Last updated:
- 6 June 2024 - 10:50am
- Document Type:
- Poster
- Document Year:
- 2024
- Event:
- Categories:
- Log in to post comments
Recent CNN and Transformer-based models tried to utilize frequency and periodicity information for long-term time series forecasting. However, most existing work is based on Fourier transform, which cannot capture fine-grained and local frequency structure. In this paper, we propose a Wavelet-Fourier Transform Network (WFTNet) for long-term time series forecasting. WFTNet utilizes both Fourier and wavelet transforms to extract comprehensive temporal-frequency information from the signal, where Fourier transform captures the global periodic patterns and wavelet transform captures the local ones. Furthermore, we introduce a Periodicity-Weighted Coefficient (PWC) to adaptively balance the importance of global and local frequency patterns. Extensive experiments on various time series datasets show that WFTNet consistently outperforms other state-of-the-art baseline. Code is available at https://github.com/Hank0626/WFTNet.
WFTNet.pdf
