Documents
Presentation Slides
WHEN VISIBLE-TO-THERMAL FACIAL GAN BEATS CONDITIONAL DIFFUSION
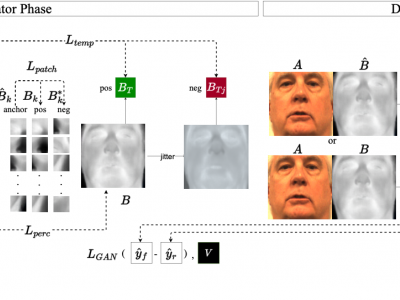
- DOI:
- 10.60864/na0j-vf20
- Citation Author(s):
- Submitted by:
- Catherine Ordun
- Last updated:
- 17 November 2023 - 12:05pm
- Document Type:
- Presentation Slides
- Document Year:
- 2023
- Event:
- Presenters:
- Catherine Ordun
- Paper Code:
- 1203
- Categories:
- Keywords:
- Log in to post comments
Thermal facial imagery offers valuable insight into physiological states such as inflammation and stress by detecting emitted radiation in the infrared spectrum, which is unseen in the visible spectra. Telemedicine applications could benefit from thermal imagery, but conventional computers are reliant on RGB cameras and lack thermal sensors. As a result, we propose the Visible-to-Thermal Facial GAN (VTF-GAN) that is specifically designed to generate high-resolution thermal faces by learning both the spatial and frequency domains of facial regions, across spectra. We compare VTF-GAN against several popular GAN baselines and the first conditional DDPM for VT face translation (VTF-Diff). Results show that VTF-GAN achieves high quality, crisp, and perceptually realistic thermal faces using a combined set of patch, temperature, perceptual, and Fourier Transform losses, compared to all baselines including diffusion.