Documents
Poster
Poster for Unsupervised User Intent Modeling by Feature-Enriched Matrix Factorization
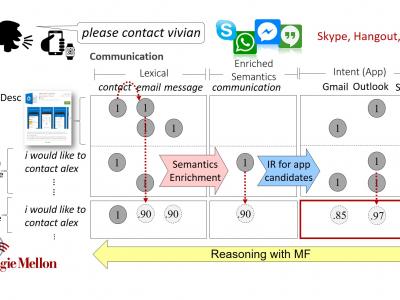
- Citation Author(s):
- Submitted by:
- Yun-Nung Chen
- Last updated:
- 31 March 2016 - 7:51pm
- Document Type:
- Poster
- Document Year:
- 2016
- Event:
- Presenters:
- Yun-Nung Chen
- Paper Code:
- HLT-P3.5
- Categories:
- Log in to post comments
Spoken language interfaces are being incorporated into various devices such as smart phones and TVs. However, dialogue systems may fail to respond correctly when users’ request functionality is not supported by currently installed apps. This paper proposes a feature-enriched matrix factorization (MF) approach to model open domain intents, which allows a system to dynamically add unexplored domains according to users’ requests. First we leverage the structured knowledge from Wikipedia and Freebase to automatically acquire domain-related semantics to enrich features of input utterances, and then MF is applied to model automatically acquired knowledge, published app textual descriptions and users’ spoken requests in a joint fashion; this generates latent feature vectors for utterances and user intents without need of prior annotations. Experiments show that the proposed MF models incorporated with rich features significantly improve intent prediction, achieving about 34% of mean average precision (MAP) for both ASR and manual transcripts.