Documents
Poster
TOTAL VARIATION REGULARIZED REWEIGHTED LOW-RANK TENSOR COMPLETION FOR COLOR IMAGE INPAINTING
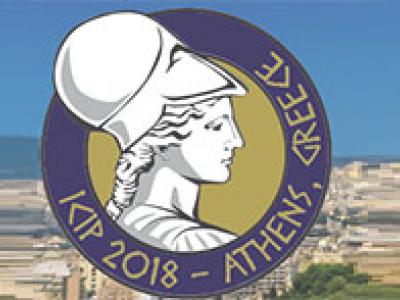
- Citation Author(s):
- Submitted by:
- Lingwei Li
- Last updated:
- 8 October 2018 - 3:01am
- Document Type:
- Poster
- Document Year:
- 2018
- Event:
- Presenters:
- Lingwei Li
- Paper Code:
- ICIP18001
- Categories:
- Log in to post comments
Recent low-rank based tensor completion (LRTC) algorithms have been successfully applied into color image inpainting. However, most of existing LRTC algorithms treat each dimension of tensors equally, which ignores the differences of the intrinsic structure correlations among dimensions. In this paper, we make a detailed analysis about the rank properties of each dimension and design a simple yet effective reweighted low-rank tensor completion model that truthfully capture the intrinsic structure correlations with reduced computational burden. Moreover, to capture the local smooth and piecewise priors of tensors, we integrate total variation into our model. Considering two formulations of LRTC, tensor unfolding and tensor decomposition, we propose corresponding two algorithms for color image recovery. Extensive experimental results on color image recovery show the efficiency and effectiveness of the proposed two algorithms against state-of-the-art competitors.
ICIP183018.pdf
