Documents
Poster
Poster
CONDITIONAL DISTRIBUTION LEARNING WITH NEURAL NETWORKS AND ITS APPLICATION TO UNIVERSAL IMAGE DENOISING
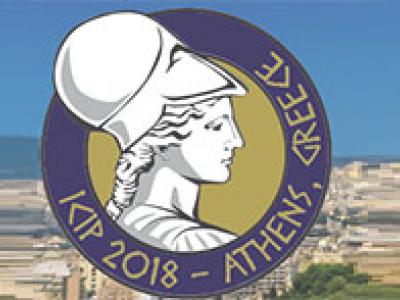
- Citation Author(s):
- Submitted by:
- Jongha Ryu
- Last updated:
- 8 October 2018 - 3:38am
- Document Type:
- Poster
- Document Year:
- 2018
- Event:
- Presenters:
- Jongha Jon Ryu
- Paper Code:
- ICIP18001
- Categories:
- Log in to post comments
A simple and scalable denoising algorithm is proposed that can be applied to a wide range of source and noise models. At the core of the proposed CUDE algorithm is symbol-by-symbol universal denoising used by the celebrated DUDE algorithm, whereby the optimal estimate of the source from an unknown distribution is computed by inverting the empirical distribution of the noisy observation sequence by a deep neural network, which naturally and implicitly aggregates multiple contexts of similar characteristics and estimates the conditional distribution more accurately. The performance of CUDE is evaluated for grayscale images of varying bit depths, which improves upon DUDE and its recent neural network based extension, Neural DUDE.