Documents
Poster
Improving Facial Attractiveness Prediction via Co-Attention Learning
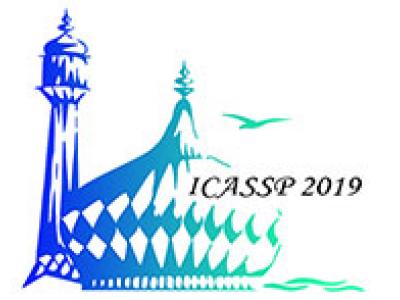
- Citation Author(s):
- Submitted by:
- Fei Gao
- Last updated:
- 7 May 2019 - 11:09pm
- Document Type:
- Poster
- Document Year:
- 2019
- Event:
- Presenters:
- Shengjie Shi
- Paper Code:
- 2862
- Categories:
- Log in to post comments
Facial attractiveness prediction has drawn considerable attention from image processing community.
Despite the substantial progress achieved by existing works, various challenges remain.
One is the lack of accurate representation for facial composition, which is essential for attractiveness evaluation. In this paper, we propose to use pixel-wise labelling masks as the meta information of facial composition, and input them into a network for learning high-level semantic representations.
The other challenge is to define to what degree different local properties contribute to facial attractiveness. To tackle this challenge, we employ a co-attention learning mechanism to concurrently characterize the significance of different regions and that of distinct facial components.
We conduct experiments on the SCUT-FBP5500 and CelebA datasets. Results show that our co-attention learning mechanism significantly improves the facial attractiveness prediction accuracy. Besides, our method consistently produces appealing results and outperforms previous advanced approaches.